Machine Learning Data Labeling: Transforming Business Operations
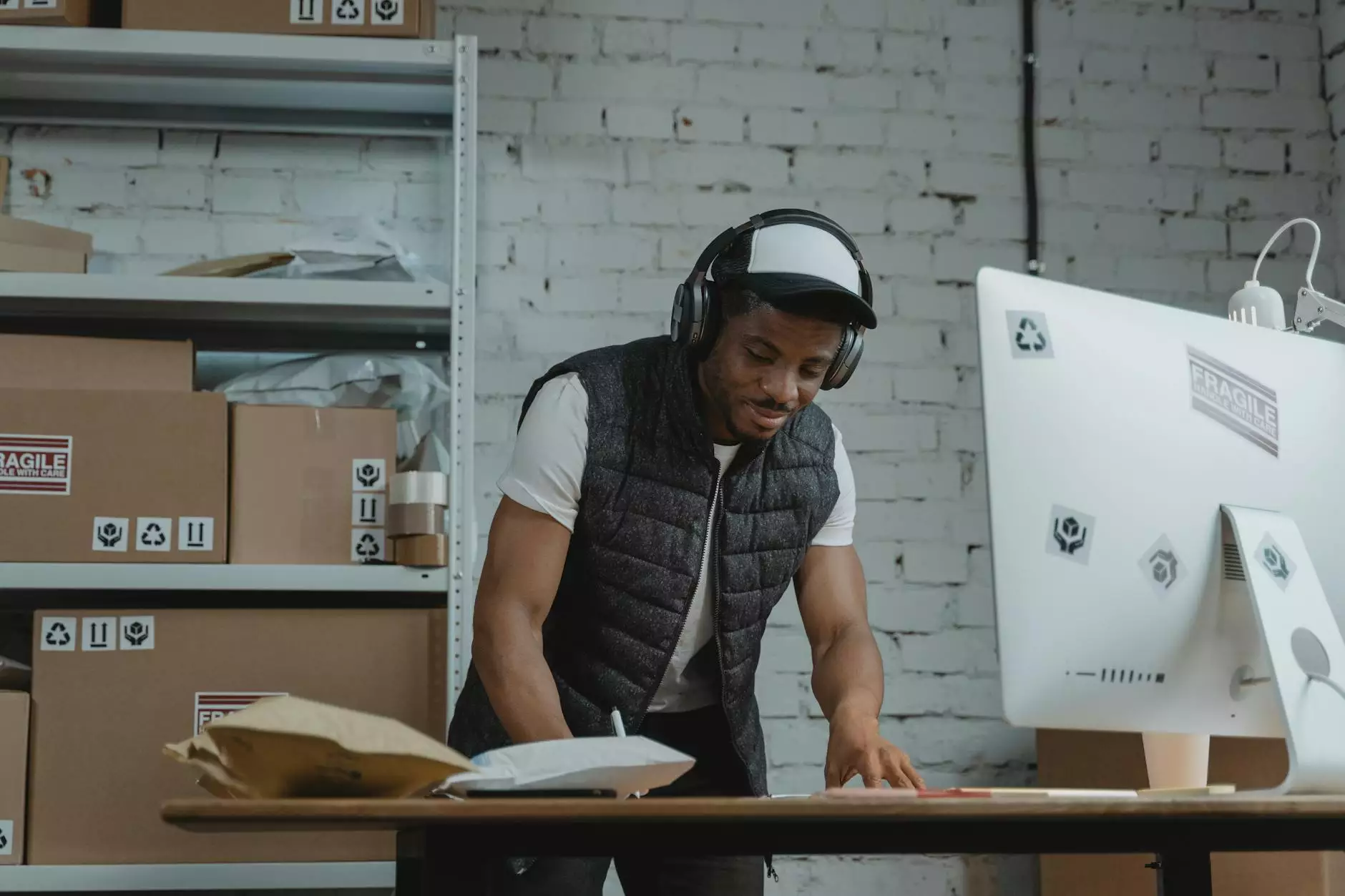
In today's fast-paced world, businesses are continuously evolving to meet customer demands and streamline operations. One of the most significant advancements driving this transformation is machine learning data labeling. This innovative approach is particularly relevant to sectors like Home Services and Keys & Locksmiths, where precision and efficiency are paramount. In this article, we will delve into the intricacies of machine learning data labeling, its applications, benefits, and how it can be leveraged to enhance business operations.
Understanding Machine Learning Data Labeling
Machine learning data labeling refers to the process of categorizing and annotating data to train machine learning models. Labeling data accurately is crucial as it directly influences the performance of AI algorithms. In essence, it involves adding meaningful tags to images, text, audio, or other types of data, enabling machines to understand and interpret information more effectively.
The Importance of Data Labeling in Business
Data is often termed as the "new oil", as it has the potential to drive significant business decisions and operations. However, raw data needs to be refined through labeling to unlock its true potential. Here’s how data labeling impacts businesses:
- Improved Decision Making: Accurate data labeling enables businesses to derive insights that lead to informed decision-making.
- Enhanced Customer Experience: By understanding customer needs through labeled data, companies can tailor their services effectively.
- Increased Operational Efficiency: Automating processes driven by machine learning reduces time and labor costs.
- Competitive Advantage: Organizations that leverage machine learning data labeling can outperform competitors by harnessing insights faster.
Applications of Machine Learning Data Labeling
Machine learning data labeling is utilized across various sectors. Here are some specific applications relevant to the home services and locksmith industries:
1. Home Services
- Image Recognition for Damage Assessment: Service providers can use machine learning models to assess property damage through labeled images.
- Lead Generation: By analyzing customer inquiries and categorizing them via data labeling, businesses can target their marketing more effectively.
- Predictive Maintenance: Data labeling helps in predicting equipment failures by analyzing historical data patterns.
2. Keys & Locksmiths
- Automated Customer Support: Chatbots can be trained on labeled data to provide accurate responses to customer queries.
- Security Analysis: Machine learning can analyze and label patterns of attempted breaches, helping locksmiths understand vulnerabilities.
- Inventory Management: Labeled data can facilitate tracking and predicting inventory needs, optimizing supply chains.
The Process of Data Labeling
The data labeling process can be complex but is generally broken down into several key steps:
- Data Collection: Amass data from relevant sources, ensuring it is diverse and represents the desired outcomes.
- Data Annotation: Use skilled labelers or automated tools to categorize data accurately according to predefined guidelines.
- Quality Assurance: Implement rigorous quality checks to ensure the labeled data meets accuracy standards.
- Model Training: Utilize the labeled data to train machine learning models and refine their algorithms.
- Ongoing Improvement: Continuously update and relabel data as needed to adapt to changing business requirements.
Challenges in Machine Learning Data Labeling
While machine learning data labeling greatly benefits businesses, it also presents several challenges:
1. Resource Intensity
Data labeling can be time-consuming and requires a significant investment in both human resources and technology.
2. Consistency and Accuracy
Ensuring consistency in labeling practices is crucial, as discrepancies can lead to bias in machine learning models.
3. Scalability
As businesses grow, the volume of data increases, making it essential to have scalable solutions for data labeling.
Best Practices for Effective Data Labeling
To overcome the challenges associated with machine learning data labeling, consider these best practices:
- Define Clear Guidelines: Establish clear and detailed labeling guidelines to ensure consistency.
- Invest in Training: Provide comprehensive training for labelers to enhance their understanding of the task.
- Utilize Automation: Implement tools and software that can automate parts of the labeling process to save time and effort.
- Regularly Review and Update: Continuously monitor the labeled data for accuracy and relevancy, making adjustments as necessary.
The Future of Machine Learning Data Labeling in Business
As we look to the future, machine learning data labeling is poised to play an even more significant role in driving business growth and innovation. The advent of more sophisticated AI technologies will lead to:
- Enhanced Automation: Greater reliance on automation will streamline the labeling process further.
- Real-time Data Processing: Businesses will increasingly use real-time data labeling to make decisions on the fly.
- Personalization: Labeled data will enable hyper-personalized customer experiences, driving loyalty and satisfaction.
- Integration with IoT: The combination of IoT devices and labeled data will result in smarter operational capabilities.
Conclusion
In conclusion, machine learning data labeling is an essential component for modern businesses striving for efficiency and excellence. By understanding its significance and implementing best practices, organizations in the home services and locksmith sectors can unlock unprecedented opportunities for growth. Embracing this technology not only enhances operational performance but also positions businesses to adapt to the ever-evolving market landscape. The future belongs to those who harness the power of data, and through effective labeling, businesses can turn information into actionable insights.